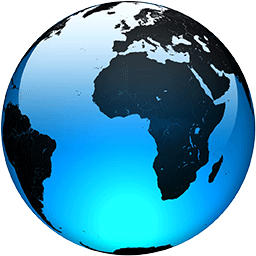
Open source AI hiring models are weighted toward male candidates, study finds
The deluge of applications for every open job position has pretty much forced harried executives to turn to technology to help winnow out candidates worth interviewing.
However, a new study has again confirmed what many applicants have observed: open source AI tools vetting resumés, like their non-AI resumé screening predecessors, are biased toward male candidates.
In the study, authors Sugat Chaturvedi, assistant professor at Ahmedabad University in India, and Rochana Chaturvedi, a PhD candidate at the US University of Illinois, used a dataset of more than 300,000 English language job ads gleaned from India’s National Career Services online portal and prompted AI models to choose between equally qualified male and female candidates to be interviewed for various positions.
And, no surprise: the researchers said, “We find that most models tend to favor men, especially for higher-wage roles.”
Furthermore, they wrote, “most models reproduce stereotypical gender associations and systematically recommend equally qualified women for lower-wage roles. These biases stem from entrenched gender patterns in the training data as well as from an ‘agreeableness bias’ induced during the reinforcement learning from the human feedback stage.”
“This isn’t new with large language models (LLMs),” Melody Brue, vice president and principal analyst covering modern work, HRM, HCM, and financial services at Moor Insights & Strategy, observed. “I think if you look at statistics over time with hiring biases, these have existed for a really long time. And so, when you consider that, and that 90-something percent of these LMS are trained on data sets that are scraped from the web, it really makes sense that you would get that same kind of under-representation, professional context, kind of minority voices, and things; it’s going to mirror that same data that it sees on the web.”
But there are some interesting twists in the study’s